A Modern Experience Management (XM) program – whether that’s a customer experience (CX) or employee experience (EX) program – needs to be capable of coordinating and consolidating the collection of all experience-related data at scale, regardless of its form or point of origin. Historically, structured, survey-based measurements have made up the backbone of these XM data collection efforts. However, thanks to several recent technological advances – such as the explosion in volume and variety of available data, increased data management and storage capabilities, and rapidly evolving Artificial Intelligence (AI) tools and Machine Learning (ML) techniques – XM teams today can capture a multitude of different data elements from a diverse set of sources.
To help XM professionals conceptualize all of the different data that they will need to work with, we’ve identified seven overlapping categories:
- Solicited Data. Solicited data is generated when an individual (customer, employee, etc.) directly responds to a specific request or prompt for feedback that is initiated by the organization. This data is often produced through surveys, forms, questionnaires, comment cards, or interviews.
- Unsolicited Data. Unsolicited data is generated when an individual voluntarily or passively provides their feedback about an organization without the organization requesting it. This data is often produced as a byproduct of other activities or interactions, such as social media posts or support conversations.
- Structured Data. Structured data is organized and formatted in a specific – often numerical – form that makes it easy to sort and analyze. It is usually housed in fixed fields within a database or spreadsheet, where each data element is labeled or categorized. Common forms of structured data include numbers, addresses, or categorical information.
- Unstructured Data. Unstructured data does not have a fixed format or organization, making it difficult to sort and analyze without preprocessing. It can take on a wide variety of different forms, including open-text, images, audio, and video.
- Behavioral Data (B-Data). Behavioral Data is derived from an individual’s actions, behaviors, or interactions. It is usually gathered through observation, tracking, or analysis of their activities, rather than provided by the individual themselves.
- Operational Data (O-Data). Operational data measures core business information such as financials, customer and employee demographics, and product ownership. It is typically derived from the day-to-day operations of an organization, often as part of transactional systems and processes.
- Experience Data (X-Data). Experience Data measures an individual’s subjective perceptions, feelings, attitudes, and expectations related to their interactions with an organization. It is provided by the individual regarding the qualitative aspects of their experience, such as their satisfaction with an interaction or how easy it was to perform a certain task.
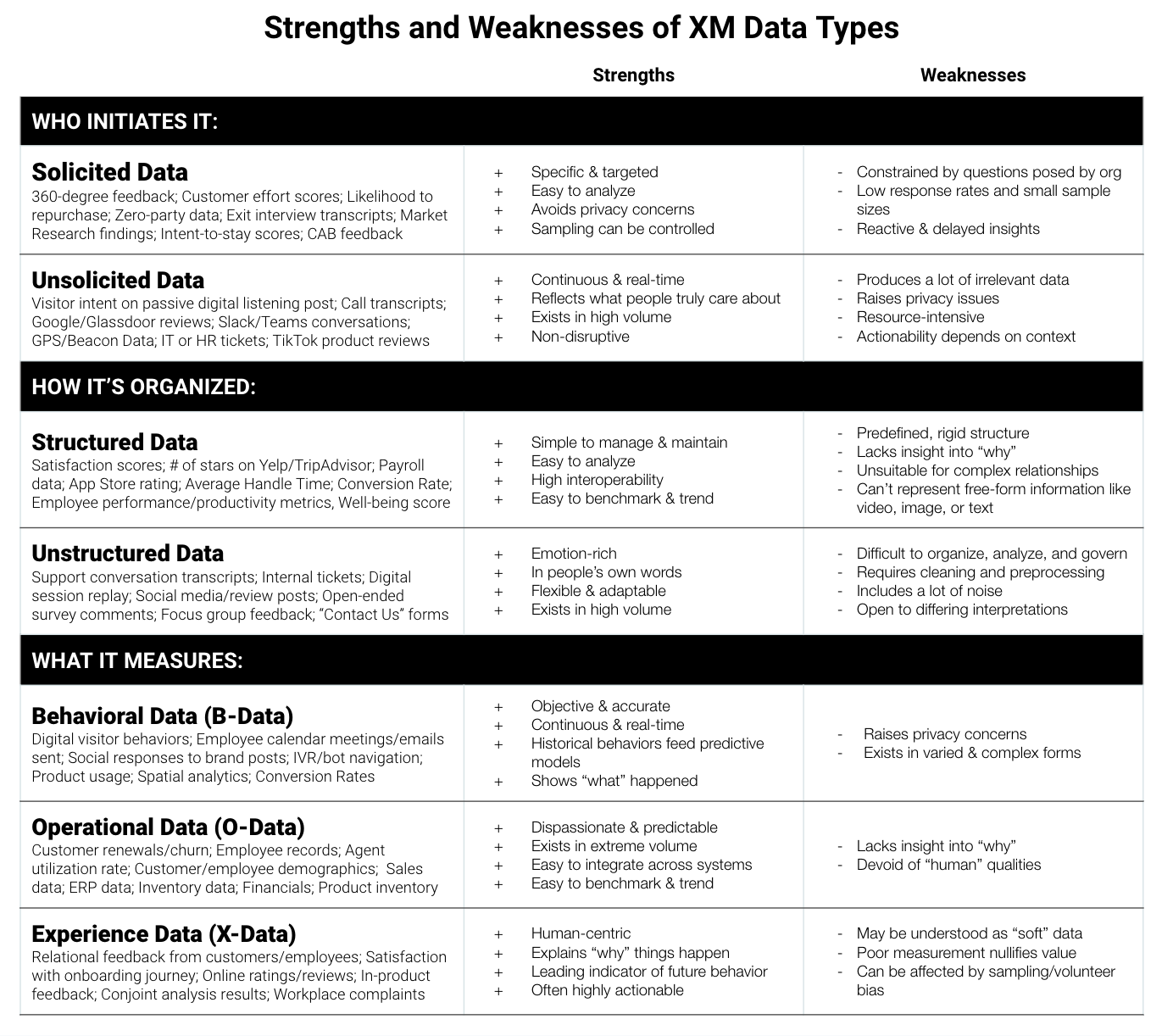
Data Elements Span Multiple Categories
These seven XM data types classify information across three different categories: who initiated the request (solicited vs. unsolicited data), how the information is organized (structured vs. unstructured data), and what is being measured (behavioral vs. operational vs. experience data). However, a single XM data element can be sorted across all three categories. For instance, an employee engagement score is an example of solicited, structured, and experience data all at once, while a session replay of a digital interaction is an example of unstructured, unsolicited, and behavioral data. Here are some common XM data elements categorized across these three areas:
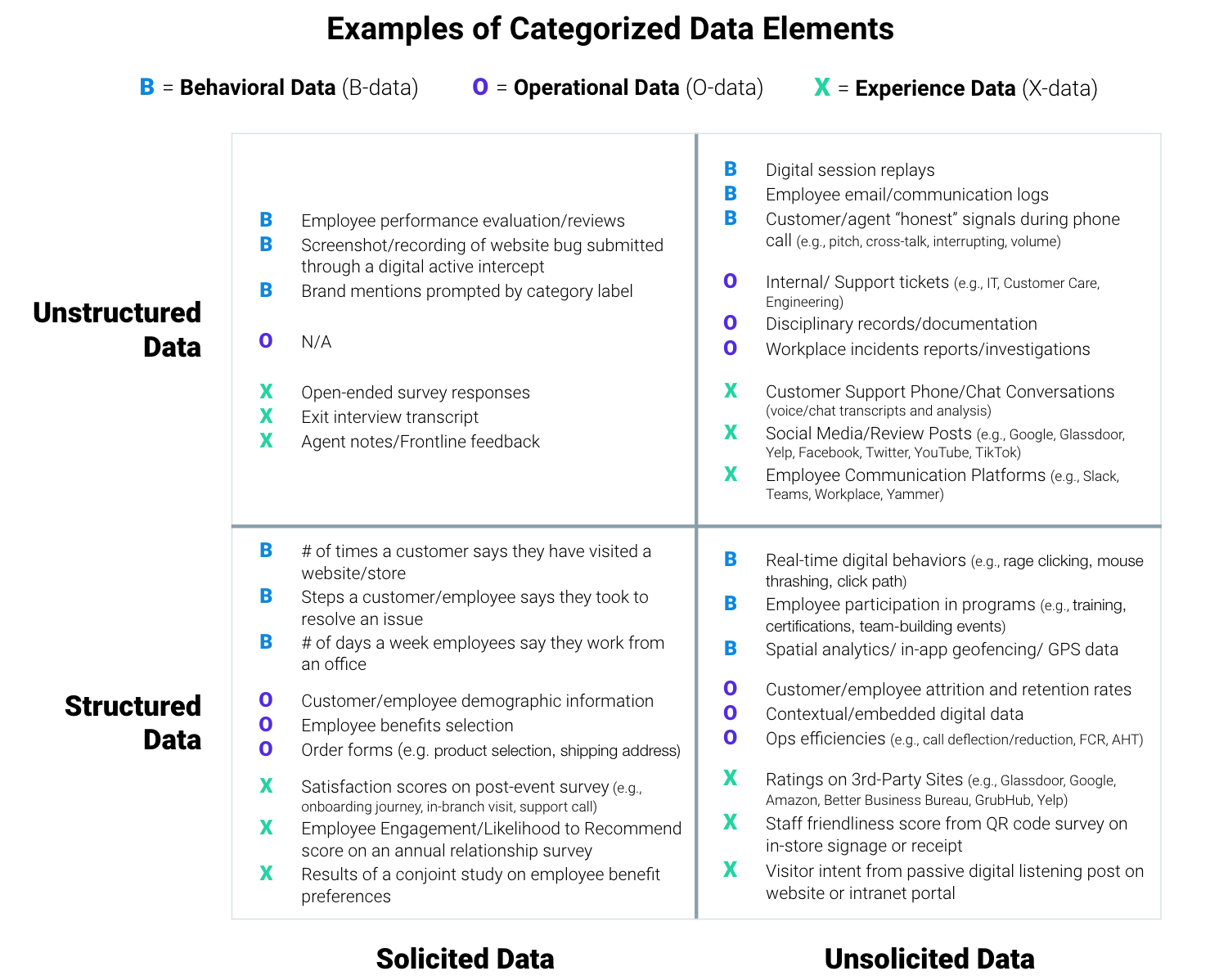
Developing Your Dynamic Instrumentation Strategy
A modern XM program adopts a hybrid approach to data collection. It dynamically instruments its listening strategy, capturing a variety of different data types, which – when taken together – produce a holistic understanding of people’s experiences with the organization. Which exact data elements are included in an XM program’s listening portfolio will depend heavily on a number of different factors, like the team’s current priorities, the organization’s broader business objectives, which industry it operates in, which use cases it is focusing on, etc. However, regardless of the specifics of your XM program, there are some general guidelines we recommend you follow to ensure you collect the right combination of data elements as part of your listening strategy:
- Define your business and strategic objectives. Rather than collecting and combining XM data randomly – which will likely result in costly and time-consuming projects that are not guaranteed to yield useful insights – start your data collection strategy by identifying the business and strategic outcomes you are looking to achieve from the data. What question are you looking to answer and what are the likely variables? For example, you might be trying to understand emerging trends in behavioral, experience, and operational (BOX) data, predict future events or behaviors, personalize experiences for specific cohorts, build a business case for XM efforts, improve word-of-mouth, or cut costs. If your objective is to improve operational efficiency by reducing the average time it takes to resolve a customer issue, for instance, you might capture operational data like average handle time and first call resolution, unstructured data from support conversations and digital help pages, and solicited post-event surveys. Starting with the end goal in mind will help you allocate and prioritize resources, avoid data overload, secure stakeholder buy-in and accountability, justify costs, and facilitate smoother data integration.
- Use a journey lens to instrument your listening posts. If you are not strategic about your data collection approach, you will likely become overwhelmed by the sheer amount of available data. To design an effective collection strategy that incorporates a variety of different data types and sources, use journey maps as a blueprint for planning out your different listening posts. For example, deploy surveys at the end of key journeys and during “moments that matter” – those moments with the highest emotional intensity – to generate targeted, specific insights about your performance during those critical episodes. Supplement that solicited feedback with additional data elements collected across the journey steps. While the exact combination of data types you collect will depend on your particular needs and capabilities, this might include things like scraping unstructured, unsolicited data from social media mentions and online reviews to better understand the awareness phase or capturing unsolicited behavioral and operational data during the usage phase.
- Harness and consolidate the data that’s already available. Designing and deploying effective surveys can take significant time and coordination. As you expand your listening portfolio, instead of creating new surveys, start by reviewing and compiling relevant data elements that already exist. This might include unstructured or unsolicited data from sources like review sites (e.g., Google, App Store, Glassdoor, Yelp), social media platforms (e.g., LinkedIn, Twitter, YouTube, Reddit), internal employee communication channels (e.g., Slack, Teams, Workplace, Yammer), and support conversations (e.g., customer service calls, IT helpdesk tickets, chat transcripts, “contact us” forms). Here you should also unify relevant BOX data that currently resides in other departments and business systems across your organization, whether that’s through connectors like APIs, applying unique identifiers, and/or investing in a single, common XM platform. Starting with the data you already have will help reduce survey fatigue, eliminate redundancies, break down silos, support more powerful analytics, and save the organization time and resources.
- Maintain a bias for unsolicited information. Today, most XM programs overly rely on solicited, survey-based feedback. While these will remain an important tool for understanding customer and employee experiences, issues like limited scope, low response rates, and data lags make them insufficient to single-handedly keep up with the ever-changing needs of an organization and the people it serves. By contrast, modern XM programs prioritize unsolicited – often unstructured – data elements, which are often much more timely and information-dense. Rather than defaulting to solicited data, you should instead collect it judiciously, keeping it reserved for the occasions when you need to produce more focused insights, understand specific populations, validate hypotheses, or train predictive models. However, a word of caution: it is essential to carefully balance data collection practices with building customer – and especially employee – trust and stay compliant with privacy regulations. Define and socialize a set of principles your organization will follow whenever capturing unsolicited data, such as pledging to communicate transparently and regularly with employees about what data you are collecting for a particular project and how exactly you intend to use that data.
- Reduce respondent burden where possible. As you develop your expanded listening strategy, consider how you can capture the insights you need to achieve your objectives while minimizing the burden on respondents. For instance, don’t ask questions you can answer through unsolicited data sources. If someone clicks multiple dead links during a digital session, you don’t then need to ask them if the site is easy to navigate. And rather than sending every customer or employee the exact same survey, ensure you only ask respondents questions that are most relevant to them by tailoring the questions, channel, and timing of your solicited feedback requests to each individual’s preference, journey, segment, and real-time behaviors. Reducing the respondent’s burden also means streamlining the number of surveys you deploy and rationalizing the number of questions you include in each one, eliminating any that you don’t intend to act on or that analyses indicate are duplicative (e.g., potentially NPS and overall satisfaction).
The bottom line: Purposefully collect all seven types of XM data to build a Modern Experience Management program.
Isabelle Zdatny, XMP, CCXP, is an XM Catalyst with Qualtrics XM Institute
Terry Anderson is a Senior Product XM Scientist with Qualtrics, specializing in Location-based CX
Laura Harding is a Product XM Scientist with Qualtrics, specializing in EX
Bruce Temkin, XMP, CCXP, is the Head of Qualtrics XM Institute